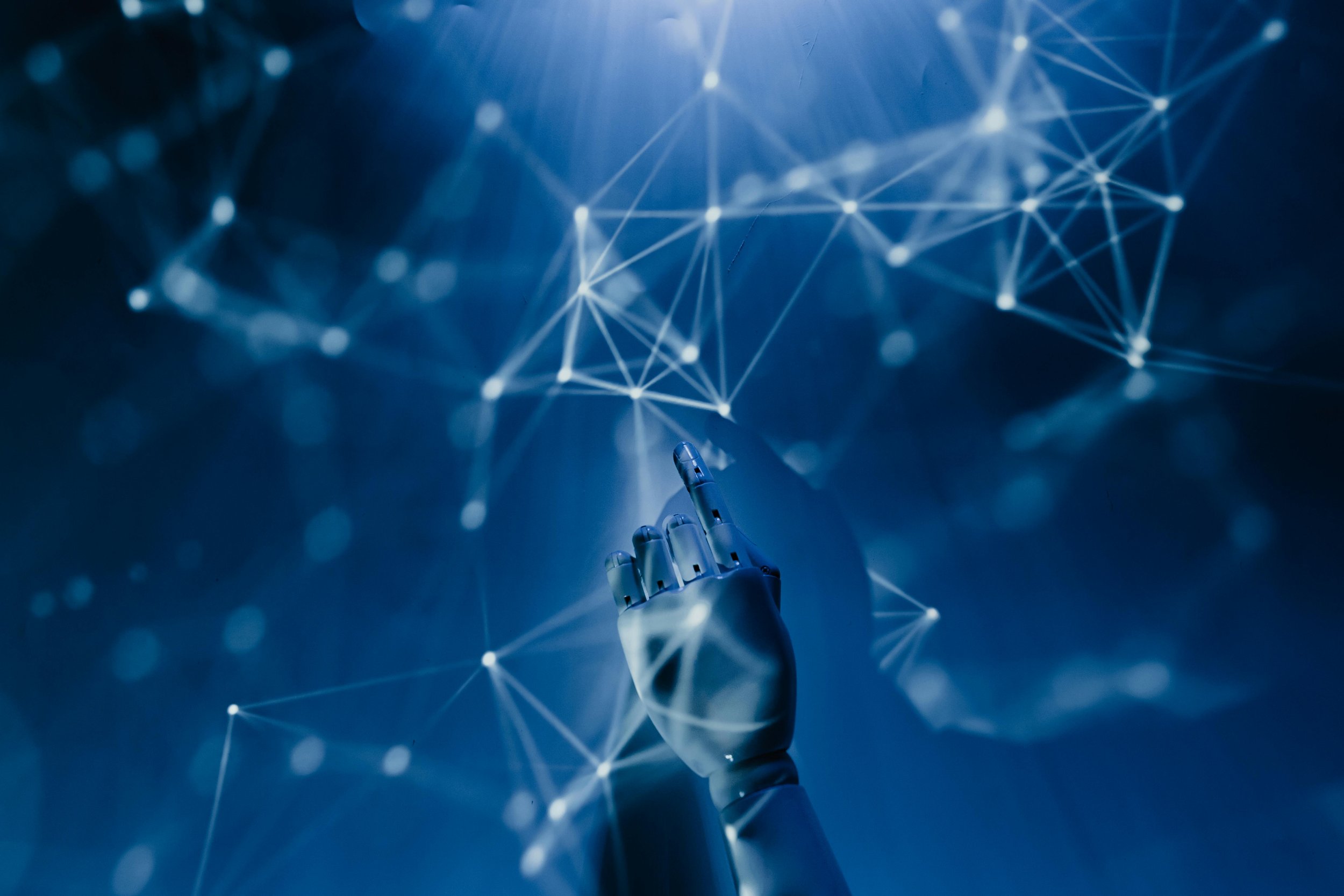
Research
Dive into my research contributions that highlight innovative applications of AI and machine learning, delivering measurable improvements in talent management, predictive maintenance, customer experience, and real-time analytics. My work has been presented at leading conferences and has driven significant advancements in AI adoption across multiple industries.
-
Conference Presentations
1. AI in HR: Leveraging Machine Learning for Talent Management
Event: HR Tech Conference, 2022
Summary: Explored the integration of AI in HR to optimize employee turnover prediction, recruitment efficiency, and performance forecasting.
Key Outcomes:
• 25% improvement in employee retention strategies using predictive analytics.
• Enhanced recruitment efficiency, reducing hiring timelines by 30%.
• Demonstrated 10% increase in workforce productivity through AI-driven performance forecasting.
Link: View Presentation on Slideshare
2. Deep Learning Models for Predictive Maintenance
Event: NeurIPS 2021
Summary: Presented a deep learning-based predictive maintenance model that reduced equipment downtime and maintenance costs.
Key Outcomes:
• 30% reduction in equipment failures through real-time failure prediction.
• Achieved 40% cost savings in maintenance operations by deploying optimized models.
• Deployed models with 95% accuracy for failure prediction in industrial settings.
Link: Read More on Slideshare
3. Enhancing Customer Experience with AI-Powered Chatbots
Event: AI Summit, 2020
Summary: Highlighted the development of NLP-driven chatbots that transformed customer support systems.
Key Outcomes:
• Improved customer satisfaction rates by 20% through enhanced interactions.
• Reduced average response times by 50%, achieving faster query resolutions.
• Successfully deployed AI chatbots for 5+ enterprises, supporting millions of user interactions monthly.
-
Conference Presentations Cont.
4. Real-Time Data Analytics with Apache Kafka and Spark
Event: Data Science Conference, 2021
Summary: Showcased scalable real-time data analytics platforms using Apache Kafka and Spark to process massive datasets efficiently.
Key Outcomes:
• Designed data pipelines that processed 1M+ events per second in real time.
• Reduced data latency by 60%, enabling near-instantaneous insights for critical business decisions.
• Improved data pipeline reliability by 40% through optimized architecture.
Link: View Presentation on Slideshare
5. Advanced Machine Learning Techniques for Financial Forecasting
Event: Financial Analytics Summit, 2021
Summary: Explored the use of machine learning for financial forecasting, enhancing accuracy in predicting market trends.
Key Outcomes:
• Improved financial forecast accuracy by 35% using time-series analysis and deep learning.
• Reduced data processing times by 25%, enabling faster decision-making for trading platforms.
• Delivered insights that drove a 15% increase in portfolio returns for clients.